We humans rely on our vision every minute and every second of the day. It's arguably one of our most important senses. So researchers started wondering, could we give computers the ability to think and see? If so, the possibilities would be endless. To answer this question, we first need to know how humans see. How do the signals from our vision process in our brains, and can they be modeled so that computers can genuinely see? These questions and the answers are at the heart of our platform. We will discover all in this blog, derived from our podcast: The Attention Podcast.
To see: a complicated process
The ability to see is a complicated cognitive process. It means you can identify the elements within your vision and translate them into thoughts and words. The process in the brain that makes all this possible is complicated, but if we understand this process, it could be modeled by a computer.
In 1950, Alan Turing, the father of theoretical computer science, was the first person to think about the question of whether or not computers could think. But we can redefine this question into ‘can computers see?’.
What could computer vision add to the real world?
If we could model how humans see and process information, we can create value for real-world scenarios. Think of how many jobs and tasks solely rely on human vision. The benefits of a computer being able to do some of that work is considerable: costs, speed, and reliability are potential gains that come to mind. One detailed example is that it can help brands create better messaging through an increased understanding of how people will see and process their messages.
Cognitive science and neuroscience are paramount to brands that make products. What we see in marketing is that the brand's message is decisive in the customer decision process; products without a resonating message are doomed. So organizations want and need to know how consumers view their message, to learn the best and most efficient way of communicating their message with their target group.
A new discovery
So how did we use computer vision to build our platform expoze.io? When the computer vision revolution was in full effect, many papers were published on object detection and salience prediction. Research found that salience could be modeled through computer vision. Salience is the information that will most likely capture one's attention in a given situation. Check the example below in figure 1.
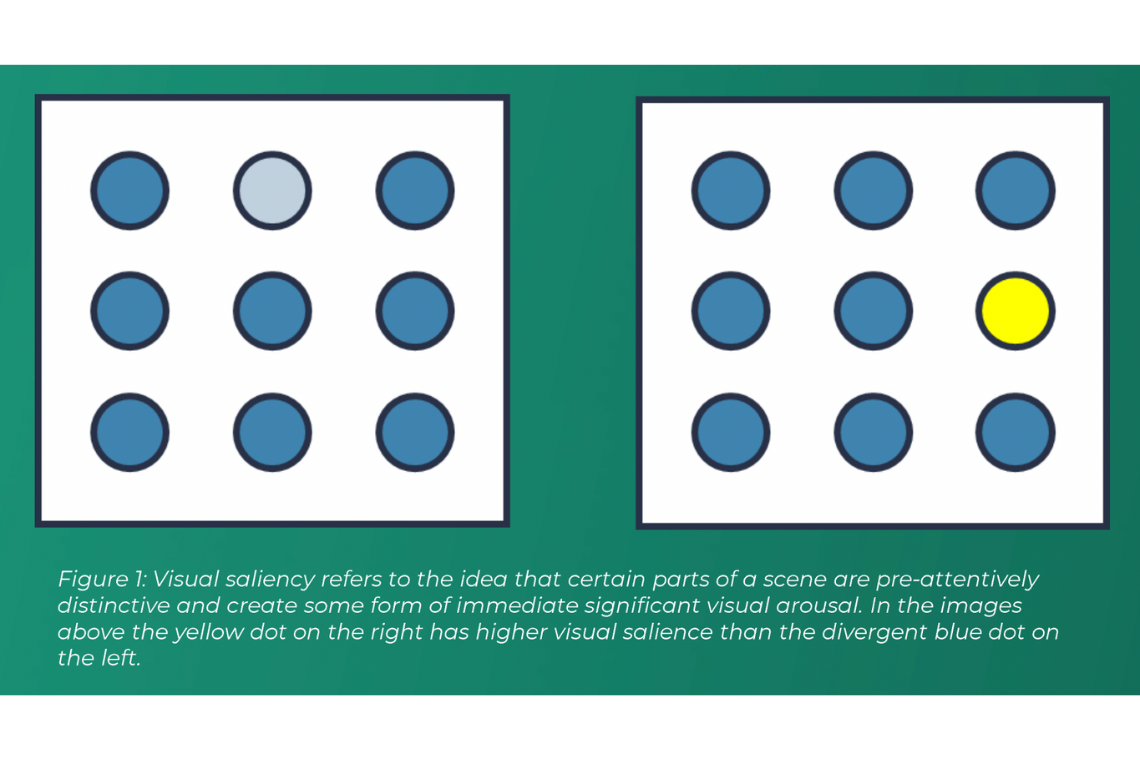
If the theory is faultless, it would mean that 100% accurate visual prediction would be possible. Test subjects undergoing eye-tracking sessions to observe marketing material could be made redundant. Eye-tracking glasses monitor your eyes through cameras and can then calculate where a person is looking within their field of view. The marketing campaign below was analyzed using traditional eye tracking with a participant.
However, knowing what a person's eyes are focusing on is not enough because the vision of a subject does not directly lead to attention and thus, perception of that subject. The brain's coping mechanism has limited processing capacity; you could technically look at something while not paying attention to it. Think of all the times you watched a video and had to rewind it because your thoughts were elsewhere, and you did not pay attention to the video.
Neural network
We started creating a neural network based on human behavioral data gathered by our parent company Alpha.One. For the use-case of a salience prediction platform like expoze.io, human data on what stands out visually. Computer vision and machine learning thrive on human data since the aim is to replicate human behavior. The more data it can learn from, the better predictions will be.
Essentially we are predicting future attention based on past attention from a colossal pool of human data. The model that learns from human data is based on neural networks. A neural network is a simulation of neuron activity in the brain created mathematically, the bigger this network is, the better the accuracy of the predictions.
The convolutional neural network
Neural networks are relatively old; they stem from the '60s. Back then, the development progress stagnated due to the lack of computing power. Simply put, the computers were not fast enough to run neural networks. However, nowadays, computers are up to the task, and in combination with a new development called a convolutional neural network, neural networks have become relevant today.
In a neural network, single neurons (transistors) are interconnected, and tens of thousands can be part of a single network. The network has to be accommodated by a computer, which causes hardware limitations.
Fortunately, activation fields were discovered, neurons are activated in large areas represented in visual patterns. Calculation of the patterns can be done efficiently through convolution, a linear algebra computation. The ability to calculate patterns of large neuron fields was a step forward over single neural processing.
So with the convolutional neural network, we try to predict visual behavioral patterns of humans and visualize them with eye tracking heatmaps. To achieve this, we gather data from a large set of visual stimuli, such as advertisements, images or video frames. The data is then exposed to a diverse group of real people to determine the salience. This data is then fed to the conventional neural network and used as training material. Find a visualization of this concept below in figure 2.
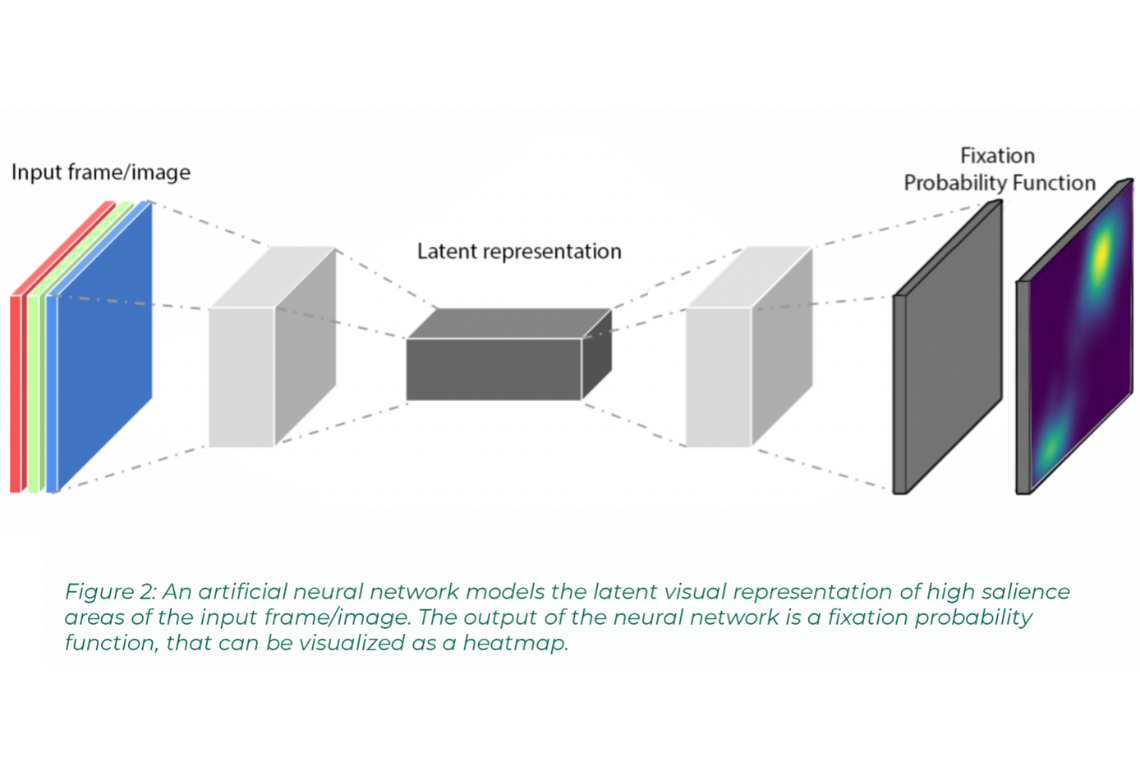
Competition makes you stronger
Furthermore, we start using a trick with a general adversarial network (GAN). It consists of two parts, the generator, and the discriminator; this couple constantly plays and learns from each other. The generator attempts to create something, let’s say: a Van Gogh painting. The discriminator then checks the painting and tells the generator it’s fake and the reasoning why. With this new information, the generator then creates a newly updated painting and returns it to the discriminator. It now has a tougher job of determining if it’s fake. This process goes on for a long time, and the two parties become better and better.
The concept behind this is game theory, a decision of one individual influences the decision of another. The GAN aims for the Nash equilibrium: at some point, the competitors have learned so much from playing that neither can improve anymore. We can measure this process to see if the maximum plateau has been achieved yet.
This entire process, as complicated as it is, has become relatively fast. Since computers have enough computing power, a revolution has started. The processing can be done on a GPU (graphics processing unit), also known as a graphics card, found in many computers. The original use case of the GPU was video gaming, but the mathematics needed for convolutional neural networks are the same as for video games, both are based on linear algebra.
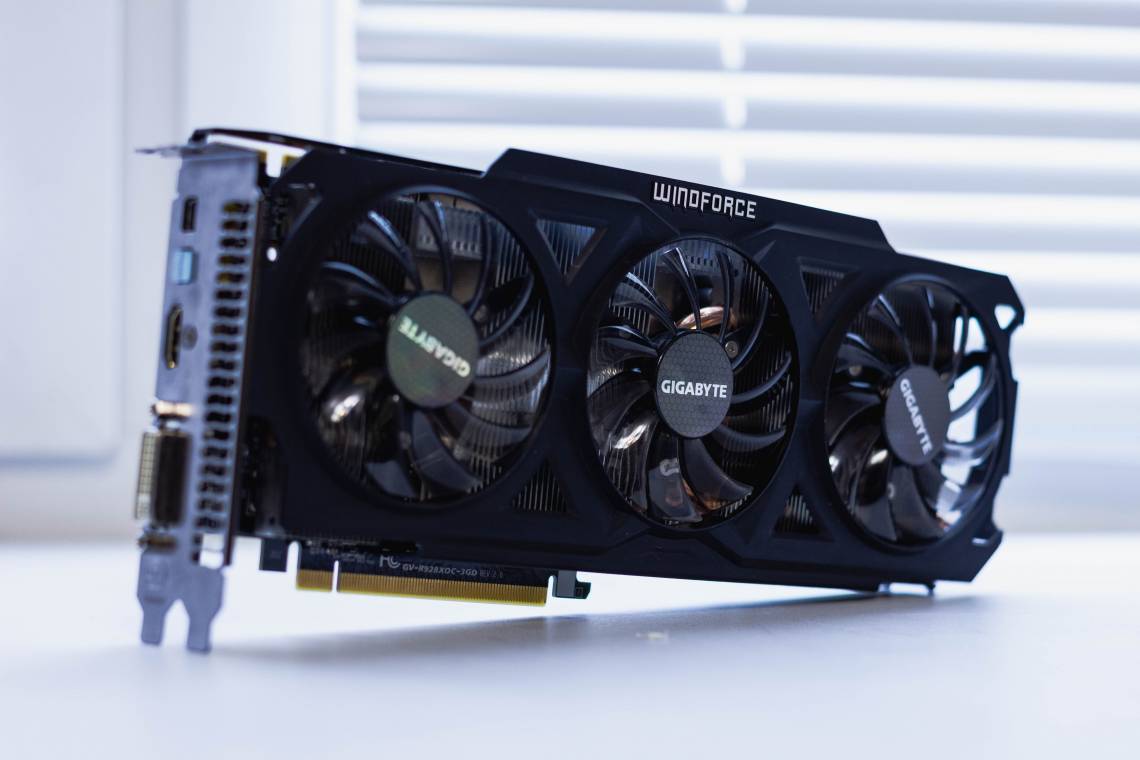
What will the future bring?
With expoze.io, we have built a platform that accurately predicts how people see things. But what more can we do in the future? What will be the limit? The neuroscience consultancy firm Alpha.One that developed expoze.io has now also created a new AI-powered platform for YouTube ad testing, called Junbi.ai. You can obtain quantified effectiveness scores and heatmaps to assess and enhance your YouTube ad in an objective manner, without the requirement for research participants or costly equipment. Check what it can do below!